![]() | This article is written like a personal reflection, personal essay, or argumentative essay that states a Misplaced Pages editor's personal feelings or presents an original argument about a topic. Please help improve it by rewriting it in an encyclopedic style. (February 2022) (Learn how and when to remove this message) |
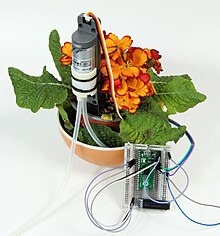
Digital agriculture, sometimes known as smart farming or e-agriculture, are tools that digitally collect, store, analyze, and share electronic data and/or information in agriculture. The Food and Agriculture Organization of the United Nations has described the digitalization process of agriculture as the digital agricultural revolution. Other definitions, such as those from the United Nations Project Breakthrough, Cornell University, and Purdue University, also emphasize the role of digital technology in the optimization of food systems.
Digital agriculture includes (but is not limited to) precision agriculture. Unlike precision agriculture, digital agriculture impacts the entire agri-food value chain — before, during, and after on-farm production. Therefore, on-farm technologies like yield mapping, GPS guidance systems, and variable-rate application, fall under the domain of precision agriculture and digital agriculture. On the other hand, digital technologies involved in e-commerce platforms, e-extension services, warehouse receipt systems, blockchain-enabled food traceability systems, tractor rental apps, etc. fall under the umbrella of digital agriculture but not precision agriculture.
Historical context
Emerging digital technologies have the potential to be game-changers for traditional agricultural practices. The Food and Agriculture Organization of the United Nations has referred to this change as a revolution: "a 'digital agricultural revolution' will be the newest shift which could help ensure agriculture meets the needs of the global population into the future." Other sources label the change as "Agriculture 4.0," indicating its role as the fourth major agricultural revolution. Precise dates of the Fourth Agricultural Revolution are unclear. The World Economic Forum announced that the "Fourth Industrial Revolution" (which includes agriculture) will unfold throughout the 21st century, so perhaps 2000 or shortly thereafter marks the beginning of Agriculture 4.0.
Agricultural revolutions denote periods of technological transformation and increased farm productivity. Agricultural revolutions include the First Agricultural Revolution, the Arab Agricultural Revolution, the British/Second Agricultural Revolution, the Scottish Agricultural Revolution, and the Green Revolution/Third Agricultural Revolution. Despite boosting agricultural productivity, past agricultural revolutions left many problems unsolved. For example, the Green Revolution had unintended consequences, like inequality and environmental damage. First, the Green Revolution exacerbated inter-farm and interregional inequality, typically biased toward large farmers with the capital to invest in new technologies. Second, critics say its policies promoted heavy input use and dependence on agrochemicals, which led to adverse environmental effects like soil degradation and chemical runoff. Digital agriculture technologies have the potential to address negative side effects of the Green Revolution.
In some ways, the Digital Agriculture Revolution follows patterns of previous agricultural revolutions. Scholars forecast a further shift away from labor, a slight shift away from capital, and intensified use of human capital — continuing the trend the British Agricultural Revolution started. Also, many predict that social backlash — possibly around the use of artificial intelligence or robots — will arise with the fourth revolution. Since controversy accompanies every societal transformation, the digital agricultural revolution isn't new in that respect.
In other ways, the Digital Agriculture Revolution is distinct from its predecessors. First, digital technologies will affect all parts of the agricultural value chain, including off-farm segments. This differs from the first three agricultural revolutions, which primarily impacted production techniques and on-farm technologies. Second, a farmer's role will require more data analytics skills and less physical interaction with livestock/fields. Third, although farming has always relied on empirical evidence, the volume of data and the methods of analysis will undergo drastic changes in the digital revolution. For example, Smart farm systems continuously monitor the behavior of your animals. Giving you insight into their behavior every moment of the day. Finally, increased reliance on big data may increase the power differential between farmers and information service providers, or between farmers and large value chain actors (like supermarkets).
Technology
Digital agriculture encompasses a wide range of technologies, most of which have multiple applications along the agricultural value chain. These technologies include, but are not limited to:
- Cloud computing/big data analysis tools
- Artificial intelligence
- Machine learning
- Distributed ledger technologies, including blockchain and smart contracts
- The Internet of Things, a principle developed by Kevin Ashton that explains how simple mechanical objects can be combined into a network to broaden understanding of that object
- Digital communications technologies, like mobile phones
- Digital platforms, such as e-commerce platforms, like bighaat, agribegri, krisikart India Provide digital information, Pesticides, agro products delivery doorstep of farmers. agro-advisory apps like plantix offers detection of crops diseases quickly which continent and economically, or e-extension website help farmers to extend their profits.
- Precision agriculture technologies, including
- Sensors, including food sensors and soil sensors
- Guidance and tracking systems (often enabled by GPS, GNSS, RFID, IoT)
- Variable-rate input technologies
- Automatic section control
- Advanced imaging technologies, including satellite and drone imagery, to look at temperature gradients, fertility gradients, moisture gradients, and anomalies in a field
- Automated machinery and agricultural robots
Effects of digital agriculture adoption
The FAO estimates the world will need to produce 56% more food (as compared to 2010, under "business as usual" growth) to feed over 9 billion in 2050. Furthermore, the world faces intersecting challenges like malnutrition, climate change, food waste, and changing diets. To produce a "sustainable food future," the world must increase food production while cutting greenhouse gas emissions and maintaining (or reducing) the land used in agriculture. Digital agriculture could address these challenges by making the agricultural value chain more efficient, equitable, and environmentally sustainable.
Efficiency
Digital technology changes economic activity by lowering the costs of replicating, transporting, tracking, verifying, and searching for data. Due to these falling costs, digital technology will improve efficiency throughout the agricultural value chain.
On-farm efficiency
On-farm, precision agriculture technologies can minimize inputs required for a given yield. For example, variable-rate application (VRA) technologies can apply precise amounts of water, fertilizer, pesticide, herbicide, etc. A number of empirical studies find that VRA improves input use efficiency. Using VRA alongside geo-spatial mapping, farmers can apply inputs to hyper-localized regions of their farm — sometimes down to the individual plant level. Reducing input use lowers costs and lessens negative environmental impacts. Furthermore, empirical evidence indicates precision agriculture technologies can increase yields. On U.S. peanut farms, guidance systems are associated with a 9% increase in yield, and soil maps are associated with a 13% increase in yield. One study in Argentina found that a precision agriculture approach based on crop physiological principles could result in 54% higher farm output.
Digital agriculture can improve the allocative efficiency of physical capital within and between farms. Often touted as "Uber for tractors," equipment-sharing platforms like Hello Tractor, WeFarmUp, MachineryLink Solutions, TroTro Tractor, and Tringo facilitate farmer rental of expensive machinery. By facilitating a market for equipment sharing, digital technology ensures fewer tractors sit idle and allows owners to make extra income. Furthermore, farmers without the resources to make big investments can better access equipment to improve their productivity.
Digital agriculture improves labor productivity through improved farmer knowledge. E-extension (electronic provision of traditional agricultural extension services) allows for farming knowledge and skills to diffuse at low cost. For example, the company Digital Green works with local farmers to create and disseminate videos about agricultural best practices in more than 50 languages. E-extension services can also improve farm productivity via decision-support services on mobile apps or other digital platforms. Using many sources of information — weather data, GIS spatial mapping, soil sensor data, satellite/drone pictures, etc. — e-extension platforms can provide real-time recommendations to farmers. For example, the machine-learning-enabled mobile app Plantix, Krisikart India diagnoses crops' diseases, pests, and nutrient deficiencies based on a smartphone photo. In a randomized control trial, Casaburi et al. (2014) found that sugarcane growers who received agricultural advice via SMS messages increased yields by 11.5% relative to the control group.
Finally, digital agriculture improves labor productivity through decreased labor requirements. Automation inherent in precision agriculture — from "milking robots on dairy farms to greenhouses with automated climate control" — can make crop and livestock management more efficient by reducing required labor.
Off-farm/market efficiency
Besides streamlining farm production, digital agriculture technologies can make agricultural markets more efficient. Mobile phones, online ICTs, e-commerce platforms, digital payment systems, and other digital agriculture technologies can mitigate market failures and reduce transaction costs throughout the value chain.
- Reducing information asymmetry: Price information affects competitive markets' efficiency because it impacts price dispersion, arbitrage, and farmer and consumer welfare. Since the marginal cost of digitally delivering information approaches zero, digital agriculture has the potential to spread price information. Aker and Fafchamps find that the introduction of mobile phone coverage in Niger reduced spatial price dispersion for agri-food products, especially for remote markets and perishable goods. Similarly, price information provided by Internet kiosks ("e-choupals") in India led to an increase in farmers' net profits as traders lost monopsony power. Other examples of digital platforms for price information include MFarm and Esoko.
- Matching buyers and sellers: E-commerce lowers the search costs of matching buyers and sellers, potentially shortening the value chain. Rather than go through dozens of intermediaries, farmers can sell directly to consumers. Market access services can also solve the matching problem without necessarily hosting online transactions. For example, Esoko sends market information (prices for specific commodities, market locations, etc.) to agents and farmers, connecting them to commodity buyers. All of these matching platforms help smallholders coordinate with buyers and enter both regional and global value chains. Finally, it's important to note that digital technologies can also facilitate matching in financial and input markets, not just producer-to-consumer output sales.
- Lowering transaction costs in commercial markets: Digital payments — whether integrated in e-commerce platforms or in mobile money accounts, e-wallets, etc. — reduce transactions costs within agricultural markets. The need for safe, rapid monetary transactions is particularly apparent in rural areas. Plus, digital payments can provide a gateway to bank accounts, insurance, and credit. Using distributed ledger technologies or smart contracts is another way to reduce trust-related transaction costs in commercial markets. Many retail and food companies have partnered with IBM to develop blockchain pilots related to food safety and traceability, and Alibaba is testing blockchain to reduce fraud in agri-food e-commerce between China and Australia/New Zealand.
- Lowering transaction costs in government services: Digital payments can also streamline government delivery of agricultural subsidies. In 2011, the Nigerian Federal Ministry of Agriculture and Rural Development started delivering fertilizer subsidy vouchers to e-wallets on mobile phones; by 2013, they had reached 4.3 million smallholders nationwide. Compared to the previous program, the e-vouchers cut costs — from 2011 to 2013, the cost per smallholder farmer receiving fertilizer went from US$225–300 to US$22. The e-vouchers also reached more smallholders, increasing from between 600,000-800,000 in 2011 to 4.3 million in 2013. In the second phase of the program, the Nigerian government developed the Nigerian Agricultural Payment Initiative (NAPI), which distributed PIN-enabled ID cards that hold subsidy information and provide access to loans and grants. Other e-wallet/e-voucher systems for agricultural subsidies exist or have been piloted in Colombia, Rwanda, Zambia, Mali, Guinea, and Niger. Besides reducing subsidy costs, governments can harness digital technology to save time. When Estonia implemented their e-ID and X-Road system, time spent applying for agricultural subsidies decreased from 300 minutes to 45 minutes per person.
Rarely does one single digital agriculture technology solve one discrete market failure. Rather, systems of digital agriculture technologies work together to solve multifaceted problems. For example, e-commerce solves two efficiency issues: difficulty matching buyers and sellers, especially in rural areas, and the high transaction costs associated with in-person, cash-based trade.
Equity
Digital agriculture shows promise for creating a more equitable agri-food value chain. Because digital technologies reduce transaction costs and information asymmetries, they can improve smallholder farmers' market access in a number of ways:
Financial inclusion
Digital agriculture technologies can expand farmers' access to credit, insurance, and bank accounts for a number of reasons. First, digital technology helps alleviate the information asymmetry that exists between farmers and financial institutions. When lenders decide a farmer's credit ceiling or insurance premium, they are usually uncertain about what risks the farmer presents. Digital technology reduces the costs of verifying farmers' expected riskiness. The Kenyan company M-Shwari uses customers' phone and mobile money records to assess creditworthiness. Organizations like FarmDrive and Apollo Agriculture incorporate satellite imagery, weather forecasts, and remote sensor data when calculating farmers' loan eligibility. Drone imagery can confirm a farmer's physical assets or land use and RFID technology allows stakeholders to monitor livestock, making it easier for insurers to understand farmers' riskiness. In all instances, low-cost digital verification reduces lenders' uncertainty: the questions "will this farmer repay the loan?" and "what risks does this farmer face?" become clearer.
Second, digital technology facilitates trust between farmers and financial institutions. A range of tools create trust, including real-time digital communication platforms and blockchain/distributed ledger technology/smart contracts. In Senegal, a digitalized, supply-chain-tracking system allows farmers to collateralize their rice to obtain the credit necessary for planting. Lenders accept rice as collateral because real-time, digital tracking assures them the product was not lost or damaged in the post-harvest process.
Market inclusion
Middlemen often extract exorbitant rents from farmers when purchasing their harvest or livestock for several reasons. First, smallholders in remote areas may be unaware of fair market prices. As a result, middlemen (who typically have better information about market conditions and prices) accrue significant market power and profits. A study conducted in the central highlands of Peru found that farmers who received market price information via mobile phone SMS increased their sales prices by 13-14% relative to farmers without access to the information. Second, smallholders produce tiny harvests compared to large producers, so they lack bargaining power with middlemen. If smallholders can aggregate or form a cooperative to sell their products together, they have more leverage. Online platforms and mobile phones can facilitate aggregation, such as Digital Green's Loop app. Third, connecting producers with final consumers can eliminate intermediaries' monopsony power, thereby raising producer profits. As mentioned above in the efficiency section, e-commerce or other market linkage platforms can connect a small farmer directly to consumers around the world.
Potential inequities resulting from digital agriculture
Though digital technologies can facilitate market access and information flow, there's no guarantee they won't exacerbate existing inequalities. Should constraints prevent a range of farmers from adopting digital agriculture, it's possible that the benefits will only accrue to the powerful.
- Large farms: When a digital agriculture technology requires much upfront investment, only large farms with sufficient assets and credit access will adopt it. For example, large farms are most likely to adopt precision agriculture technologies because of high costs. Increasingly however, automated mechanization is focusing on more but smaller autonomous machines, instead of fewer but larger machines such as observed with machines that still require human control. This trend enables smaller farms to participate in digital agriculture more evenly with larger farms, as the upfront investment becomes more equal relative to the size of the farm.
- Digital divide: The uneven access to information and communication technologies (ICTs) may lead to uneven adoption of — and thereby uneven gains from — digital agriculture. When digital technologies require specific skills, benefits may accrue to digitally literate farmers positioned to take advantage of such opportunities.
- Gender: Given gender-based disparities in ICT access and the gender gap in agribusiness value chains, men seem more likely to adopt digital agriculture. Therefore, digital technologies could perpetuate gender inequalities in the agricultural sector.
- Unskilled labor: Advances in on-farm productivity, particularly through digitized automation and precision agriculture, may threaten low-skilled jobs. According to the OECD, agriculture will be one of the sectors most affected by automation and McKinsey Global Institute projects that automation will displace 15% of agricultural workers in Mexico and 30% in Germany.
- Agribusinesses and service providers: Increased reliance on big data may increase the power differential between agribusinesses/information service providers and farmers. If smallholders lack access to and/or control of their data, they may lose bargaining power vis-à-vis large value chain actors (like supermarkets) and data collectors.
Environment
Boosting natural resource efficiency is the "single most important need for a sustainable food future," according to the World Resource Institute. As mentioned in the on-farm efficiency section, precision farming — including variable rate nutrient application, variable rate irrigation, machine guidance, and variable rate planting/seeding — could minimize use of agricultural inputs for a given yield. This could mitigate resource waste and negative environmental externalities, like greenhouse gas (GHG) emissions, soil erosion, and fertilizer runoff. For example, Katalin et al. 2014 estimate that switching to precision weed management could save up to 30,000 tons of pesticide in the EU-25 countries. González-Dugo et al. 2013 found that precision irrigation of a citrus orchard could reduce water use by 25 percent while maintaining a constant yield. Basso et al. 2012 demonstrated that variable-rate application of fertilizer can reduce nitrogen application and leaching without affecting yield and net return.
However, precision agriculture could also accelerate farms' depletion of natural resources because of a rebound effect; increasing input efficiency does not necessarily lead to resource conservation. Also, by changing economic incentives, precision agriculture may hinder environmental policies' effectiveness: "Precision agriculture can lead to higher marginal abatement costs in the form of forgone profits, decreasing producers' responsiveness to those policies." In other words, holding pollution constant, precision agriculture allows a farmer to produce more output — thus, abatement becomes more expensive.
Off-farm, digital agriculture has the potential to improve environmental monitoring and food system traceability. The monitoring costs of certifying compliance with environmental, health, or waste standards are falling because of digital technology. For example, satellite and drone imagery can track land use and/or forest cover; distributed ledger technologies can enable trusted transactions and exchange of data; food sensors can monitor temperatures to minimize contamination during storage and transport. Together, technologies like these can form digital agriculture traceability systems, which allow stakeholders to track agri-food products in near-real-time. Digital traceability yields a number of benefits, environmental and otherwise:
- Reduced food waste: Of all the food calories produced in a year, 25% are wasted between on-farm production and consumers. Traceability systems facilitate better identification of supply-side weaknesses — where food is lost downstream of the farm, and how much is wasted. Emerging digital innovations, such as milk cartons that track milk from "farm to fridge," can address demand-side waste by providing consumers with more accurate expiration dates.
- Consumer trust: Ensuring food safety, quality, and authenticity has become an important regulatory requirement in high-income countries. Use of RFID tags and blockchain technologies to certify agri-food products' characteristics could provide near-real-time quality signals to consumers.
- Improved producer welfare: Producers who can leverage environmental certification could sell their products at a premium, because blockchain technologies could enable greater trust in labels like "sustainable," "organic" or "fair trade."
Enabling environment
According to the McKinsey Industry Digitization Index, the agricultural sector is the slowest adopter of digital technologies in the United States. Farm-level adoption of digital agriculture varies within and between countries, and uptake differs by technology. Some characterize precision agriculture uptake as rather slow. In the United States in 2010-2012, precision agriculture technologies were used on 30-50% of corn and soybean acreage. Others point out that uptake varies by technology — farmer use of GNSS guidance has grown rapidly, but variable-rate technology adoption rarely exceeds 20% of farms. Furthermore, digital agriculture is not limited to on-farm precision tools, and these innovations typically require less upfront investment. Growing access to ICTs in agriculture and a booming e-commerce market all bode well for increased adoption of digital agriculture downstream of the farm.
Individual farmers' perceptions about usefulness, ease of use, and cost-effectiveness impact the spread of digital agriculture. In addition, a number of broader factors enable the spread of digital agriculture, including:
Digital infrastructure
Although a few digital technologies can operate in areas with limited mobile phone coverage and internet connectivity, rural network coverage plays an important role in digital agriculture's success. A wide gap exists between developed and developing countries' 3G and 4G cellular coverage, and issues like dropped calls, delays, weak signals, etc. hamper telecommunications efficacy in rural areas. Even when countries overcome infrastructural challenges, the price of network connectivity can exclude smallholders, poor farmers, and those in remote areas. Similar accessibility and affordability issues exist for digital devices and digital accounts. According to a 2016 GSMA report, of the 750 million-plus farmers in the 69 surveyed countries, about 295 million had a mobile phone; only 13 million had both a mobile phone and a mobile money account. Despite lingering gaps in network coverage, ICT access has skyrocketed in recent years. In 2007, only 1% of people in developing countries used Internet, but by 2015, 40% did. Mobile-broadband subscriptions, which increased thirty-fold between 2005 and 2015, drove much of this growth. As a key enabler of agricultural change, digital infrastructure requires further development, but growing ICT access indicates progress.
Agriculture's role in the economy
The significance and structure of a country's agricultural sector will affect digital agriculture adoption. For example, a grain-based economy needs difference technologies than a major vegetable producer. Automated, digitally-enabled harvesting systems might make sense for grains, pulses and cotton, but only a few specialty crops generate enough value to justify large investments in mechanized or automated harvesting. Farm size also affects technology choices, as economies of scale make large investments possible (e.g., adoption of precision agriculture is more likely on larger farms). On the other hand, digital agriculture solutions focused on ICTs and e-commerce would benefit an economy dominated by smallholders. In China, where the average farm size is less than 1 ha, Alibaba's customer-to-customer e-commerce platform called Rural Taobao has helped melon growers in Bachu County market their produce all over the country. Other structural factors, such as percent of the population employed in agriculture, farm density, farm mechanization rates, etc. also impact how difference regions adopt digital agriculture.
Human capital
In order to benefit from the advent of digital agriculture, farmers must develop new skills. As Bronson (2018) notes, "training a rural work-force in Internet technology skills (e.g., coding) is obviously a key part of agricultural "modernization." Integration into the digital economy requires basic literacy (ability to read) and digital literacy (ability to use digital devices to improve welfare). In many instances, benefiting from digital content also requires English literacy or familiarity with another widely spoken language. Digital agriculture developers have designed ways around these barriers, such as ICTs with audio messages and extension videos in local languages. However, more investment in human capital development is needed to ensure all farmers can benefit from digital agriculture.
Fostering human capital in the form of innovation also matters for the spread of digital agriculture. Some characterize digital agriculture innovation, a knowledge- and skills-intensive process, as concentrated in "Big Ag" companies and research universities. However, others describe small-scale entrepreneurs as the "heart of the action." In 2018, ag-tech innovation attracted $1.9 billion in venture capital, and the sector has grown significantly in the last 10 years. Although digital agriculture may be concentrated in a few developed countries because of "structure, institutional, and economic barriers," ag-tech startups have experienced significant growth in Africa, the Caribbean and Pacific, Asia, and Latin America as well.
Policy and regulatory environment
In order for digital agriculture to spread, national governments, multilateral organizations, and other policymakers must provide a clear regulatory framework so that stakeholders feel confident investing in digital agriculture solutions. Policy designed for the pre-Internet era prevents the advancement of "smart agriculture," as does regulatory ambiguity. Furthermore, a blurry line between personal and business data when discussing family farms complicates data regulation. The unanswered regulatory questions mostly concern big data, and they include:
- Ensuring data privacy and security: Farmers have concerns about who can access their data. Their concerns extend to government use of data; German farmers reported a "lack of data security and excessive transparency vis-à-vis the public authorities." Scholars have issued repeated calls for policymakers to address agricultural data privacy and security.
- Address data ownership: According to the European Parliamentary Research Service, "it is clear that the farmer owns the data generated on his fields." The German Agricultural Society and others concur. However, in practice, farmers lack control over data about themselves and their farms.
Besides establishing regulations to boost stakeholder confidence, policymakers can harness digital agriculture for the provision of public goods. First, the United Nations' Global Open Data for Agriculture and Nutrition (GODAN) calls for open access to agricultural data as a basic right. Rather than stakeholders operating in "data silos" — where no one shares information for fear of competition — open data sources (when appropriately anonymized) can foster collaboration and innovation. Open-sourced data can rebalance the power asymmetry between farmers and large agribusinesses who collect data. Second, governments can finance research and development of digital agriculture. For big data analytics tools "to enter the public domain, work for the common good and not just for corporate interests, they need to be funded and developed by public organizations." The United Kingdom, Greece, and other national governments have already announced large investments in digital agriculture. Governments can also engage in private-public R&D partnerships to foster smallholder-oriented digital agriculture projects in developing countries. Lastly, digital agriculture technologies — particularly traceability systems — can improve monitoring of environmental compliance, evaluation of subsidy eligibility, etc.
Finally, when governments and international undertake complementary investments, they can strengthen the enabling environment for digital agriculture. By improving digital infrastructure, choosing digital agriculture technologies appropriate for the regional context, and investing in human capital/digital skills development, policymakers could support digital agriculture.
Research environment
In the United States, research in digital agriculture is primarily funded by the National Institute of Food and Agriculture (NIFA) which comes under the US Department of Agriculture and to a lesser extent, by the National Science Foundation. Two large institutes applying IoT or artificial intelligence in digital agriculture have been unveiled by these funding organizations working together.
- iot4Ag: The Internet of Things for Precision Agriculture an NSF Engineering Research Center
- COALESCE: COntext-Aware LEarning for Sustainable CybEr-agricultural systems
Sustainable Development Goals
According to Project Breakthrough, digital agriculture can help advance the United Nations Sustainable Development Goals by providing farmers with more real-time information about their farms, allowing them to make better decisions. Technology allows for improved crop production by understanding soil health. It allows farmers to use fewer pesticides on their crops. Soil and weather monitoring reduces water waste. Digital agriculture ideally leads to economic growth by allowing farmers to get the most production out of their land. The loss of agricultural jobs can be offset by new job opportunities in manufacturing and maintaining the necessary technology for the work. Digital agriculture also enables individual farmers to work in concert, collecting and sharing data using technology. and The hope is that young people want to become digital farmers
References
- "Technology and digital in agriculture - OECD". www.oecd.org. Retrieved 2019-07-25.
- ^ Trendov, Nikola M.; Varas, Samuel; Zeng, Meng. "Digital Technologies in Agriculture and Rural Areas" (PDF). Retrieved 17 October 2021.
- "Digital Agriculture: feeding the future". Project Breakthrough. Retrieved 2019-07-25.
- "Digital Agriculture". Cornell University. Retrieved 2019-07-25.
- "Home". Purdue University Digital Agriculture. Retrieved 2019-07-25.
- ^ Shepherd, Mark; Turner, James A.; Small, Bruce; Wheeler, David (2018). "Priorities for science to overcome hurdles thwarting the full promise of the 'digital agriculture' revolution". Journal of the Science of Food and Agriculture. 100 (14): 5083–5092. doi:10.1002/jsfa.9346. PMC 7586842. PMID 30191570.
- Rose, David Christian; Chilvers, Jason (2018). "Agriculture 4.0: Broadening Responsible Innovation in an Era of Smart Farming". Frontiers in Sustainable Food Systems. 2: 87. doi:10.3389/fsufs.2018.00087.
- Schwab, Karl (2018). The Fourth Industrial Revolution. Crown Publishing Group.
- Schwab 2018. The Fourth Industrial Revolution. Encyclopedia Britannica. https://www.britannica.com/topic/The-Fourth-Industrial-Revolution-2119734 .
- Allen, Robert C. (1999). "Tracking the agricultural revolution in England". The Economic History Review. 52 (2): 209–235. doi:10.1111/1468-0289.00123.
- ^ Freebairn (1995). "Did the Green Revolution Concentrate Incomes? A Quantitative Study of Research Reports". World Development. 23 (2): 265–279. doi:10.1016/0305-750X(94)00116-G.
- Junankar, P. N. (1975). "Green Revolution and Inequality". Economic and Political Weekly. 10 (13): A15 – A18. ISSN 0012-9976. JSTOR 4536986.
- Pingali, P. L. (2012). "Green Revolution: Impacts, limits, and the path ahead". Proceedings of the National Academy of Sciences of the United States of America. 109 (31): 12302–12308. Bibcode:2012PNAS..10912302P. doi:10.1073/pnas.0912953109. PMC 3411969. PMID 22826253.
- Food and Agriculture Organization of the United Nations. "Crop breeding: the Green Revolution and the preceding millennia". FAO Newsroom. Archived from the original on 2021-11-08. Retrieved 2019-07-26.
- Struik and Kuyper (2017). "Sustainable intensification in agriculture: the richer shade of green. A review". Agronomy for Sustainable Development. 37 (5): 37–39. doi:10.1007/s13593-017-0445-7.
- ^ Bronson (2018). "Smart Farming: Including Rights Holders for Responsible Agricultural Innovation". Technology Innovation Management Review. 8 (2). doi:10.1007/s13593-017-0445-7.
- Rose, David Christian; Chilvers, Jason (2018). "Agriculture 4.0: Broadening Responsible Innovation in an Era of Smart Farming". Frontiers in Sustainable Food Systems. 2. doi:10.3389/fsufs.2018.00087.
- MacNaghten, Phil (2015). "A Responsible Innovation Governance Framework for GM Crops". Governing Agricultural Sustainability. pp. 225–239. doi:10.4324/9781315709468-19. ISBN 978-1-315-70946-8.
- MacNaghten, Phil; Chilvers, Jason (2014). "The Future of Science Governance: Publics, Policies, Practices". Environment and Planning C: Government and Policy. 32 (3): 530–548. Bibcode:2014EnPlC..32..530M. doi:10.1068/c1245j. S2CID 144164733.
- Hartley, Sarah; Gillund, Frøydis; Van Hove, Lilian; Wickson, Fern (2016). "Essential Features of Responsible Governance of Agricultural Biotechnology". PLOS Biology. 14 (5): e1002453. doi:10.1371/journal.pbio.1002453. PMC 4856357. PMID 27144921.
- ^ Wolfert, Sjaak; Ge, Lan; Verdouw, Cor; Bogaardt, Marc-Jeroen (1 May 2017). "Big Data in Smart Farming – A review". Agricultural Systems. 153: 69–80. Bibcode:2017AgSys.153...69W. doi:10.1016/j.agsy.2017.01.023. ISSN 0308-521X.
- ^ Eastwood, C.; Klerkx, L.; Ayre, M.; Dela Rue, B. (26 December 2017). "Managing Socio-Ethical Challenges in the Development of Smart Farming: From a Fragmented to a Comprehensive Approach for Responsible Research and Innovation". Journal of Agricultural and Environmental Ethics. 32 (5–6): 741–768. doi:10.1007/s10806-017-9704-5. ISSN 1187-7863.
- Carolan, Michael (2017). "Publicising Food: Big Data, Precision Agriculture, and Co-Experimental Techniques of Addition: Publicising Food". Sociologia Ruralis. 57 (2): 135–154. doi:10.1111/soru.12120.
- Driessen, Clemens; Heutinck, Leonie F. M. (2015). "Cows desiring to be milked? Milking robots and the co-evolution of ethics and technology on Dutch dairy farms". Agriculture and Human Values. 32 (1): 3–20. doi:10.1007/s10460-014-9515-5. ISSN 0889-048X. S2CID 154358749.
- Holloway, Lewis; Bear, Christopher (2017). "Bovine and human becomings in histories of dairy technologies: robotic milking systems and remaking animal and human subjectivity" (PDF). BJHS Themes. 2: 215–234. doi:10.1017/bjt.2017.2. ISSN 2058-850X.
- Wolf, S.A.; Wood, S.D. (1997). "Precision farming: environmental legitimation, commodification of information, and industrial coordination". Rural Sociology. 62 (2): 180–206. doi:10.1111/j.1549-0831.1997.tb00650.x.
- "Smart farming: a revolutionary system by Fancom for farmers". Fancom BV. Retrieved 19 November 2020.
- ^ Carbonell (2016). "The ethics of big data in agriculture". Internet Policy Review. 5 (1). doi:10.14763/2016.1.405.
- Gabbai, Arik. "Kevin For example, Ashton Describes "The Internet of Things"". Smithsonian. Retrieved 9 December 2018.
- Zhang, Chunhua; Kovacs, John M. (31 July 2012). "The application of small unmanned aerial systems for precision agriculture: a review". Precision Agriculture. 13 (6): 693–712. Bibcode:2012PrAgr..13..693Z. doi:10.1007/s11119-012-9274-5. S2CID 14557132.
- FAO 2017. The Future of Food and Agriculture: Trends and Challenges. Rome. Accessed 11 July 2019. http://www.fao.org/3/a-i6583e.pdf .
- "Insights: WRI's Blog". World Resources Institute. Archived from the original on December 5, 2018. Retrieved 26 July 2019.
- H. Charles J. Godfray; John R. Beddington; Ian R. Crute; Lawrence Haddad; David Lawrence; James F. Muir; Jules Pretty; Sherman Robinson; Sandy M. Thomas; Camilla Toulmin (2010). "Food Security: The Challenge of Feeding 9 Billion People". Science. 327 (5967): 812–818. Bibcode:2010Sci...327..812G. doi:10.1126/science.1185383. PMID 20110467.
- ^ Searchinger, Timothy D. (19 July 2019). Creating a Sustainable Food Future. World Resources Institute. ISBN 978-1-56973-963-1. Retrieved 26 July 2019.
- Goldfarb and Tucker (2017). "Digital Economics". National Bureau of Economic Research. Working Paper No. 23684.
- Stamatiadis (EU Project Manager) 2013. "HydroSense – Innovative precision technologies for optimized irrigation and integrated crop management in a water-limited agrosystem." http://ec.europa.eu/environment/life/project/Projects/index.cfm?fuseaction=search.dspPage&n_proj_id=3466&docType=pdf .
- Tekin (2010). "Variable rate fertilizer application in Turkish wheat agriculture: Economic assessment". African Journal of Agricultural Research. 5 (8): 647–652.
- Biggar et al. 2013. "Greenhouse Gas Mitigation Options and Costs for Agricultural Land and Animal Production within the United States." ICF International – Report for USDA.
- ^ Pedersen, Søren Marcus; Lind, Kim Martin, eds. (2017). Precision Agriculture: Technology and Economic Perspectives. Progress in Precision Agriculture. doi:10.1007/978-3-319-68715-5. ISBN 978-3-319-68713-1. ISSN 2511-2260. S2CID 8032908.
- Saavoss, Monica (2018). "Productivity and profitability of precision agriculture technologies on peanut farms". USDA Economic Research Service.
- Ortiz, B. V.; Balkcom, K. B.; Duzy, L.; van Santen, E.; Hartzog, D. L. (1 August 2013). "Evaluation of agronomic and economic benefits of using RTK-GPS-based auto-steer guidance systems for peanut digging operations". Precision Agriculture. 14 (4): 357–375. Bibcode:2013PrAgr..14..357O. doi:10.1007/s11119-012-9297-y. ISSN 1573-1618. S2CID 15563611.
- Monzon, J. P.; Calviño, P. A.; Sadras, V. O.; Zubiaurre, J. B.; Andrade, F. H. (1 September 2018). "Precision agriculture based on crop physiological principles improves whole-farm yield and profit: A case study". European Journal of Agronomy. 99: 62–71. Bibcode:2018EuJAg..99...62M. doi:10.1016/j.eja.2018.06.011. hdl:11336/85293. ISSN 1161-0301. S2CID 92102740.
- Diaz, Joy (29 March 2016). "Meet A Tractor That Can Plow Fields And Talk To The Cloud". NPR.org. Retrieved 26 July 2019.
- "Hello Tractor Site". Hello Tractor. Retrieved 21 October 2020.
- "Agriculture and food: the rise of digital platforms". Paris Innovation Review. Retrieved 26 July 2019.
- "Location et Prestation de matériels agricoles - WeFarmUp". www.wefarmup.com (in French). Retrieved 26 July 2019.
- Zuckerman, Jake (22 June 2016). "Machinery Link: Where Uber meets agriculture". The Northern Virginia Daily. Retrieved 26 July 2019.
- Vota, Wayan (31 May 2017). "Uber for Tractors is Really a Thing in Developing Countries". ICTworks. Retrieved 26 July 2019.
- ^ ICT in Agriculture (Updated ed.). World Bank. 27 June 2017. doi:10.1596/978-1-4648-1002-2. hdl:10986/27526. ISBN 978-1-4648-1002-2.
- ^ "Videos". Digital Green. Retrieved 26 July 2019.
- ^ "The Future of Food: Harnessing Digital Technologies to Improve Food System Outcomes". World Bank. 2019. doi:10.1596/31565. S2CID 29071231.
- Casaburi et al. 2014. "Harnessing ICT to Increase Agricultural Production: Evidence from Kenya."
- "Digital Agriculture". Cornell University. Retrieved 26 July 2019.
- Morgan-Davies, Claire; Lambe, Nicola; Wishart, Harriet; Waterhouse, Tony; Kenyon, Fiona; McBean, Dave; McCracken, Davy (1 February 2018). "Impacts of using a precision livestock system targeted approach in mountain sheep flocks". Livestock Science. 208: 67–76. doi:10.1016/j.livsci.2017.12.002. ISSN 1871-1413.
- ^ Seabrook, John (8 April 2019). "The Age of Robot Farmers". The New Yorker. ISSN 0028-792X. Retrieved 26 July 2019.
- Fafchamps, Marcel; Aker, Jenny C. (1 January 2015). "Mobile Phone Coverage and Producer Markets: Evidence from West Africa" (PDF). The World Bank Economic Review. 29 (2): 262–292. doi:10.1093/wber/lhu006. hdl:10986/25842. ISSN 0258-6770.
- ^ Goyal, Aparajita (2010). "Information, Direct Access to Farmers, and Rural Market Performance in Central India" (PDF). American Economic Journal: Applied Economics. 2 (3): 22–45. doi:10.1257/app.2.3.22. ISSN 1945-7782. JSTOR 25760218. S2CID 54019597.
- Andres, Dustin (20 July 2012). "ICT Innovations: with Mfarm, agribusiness meets the app economy in Kenya". USAID Feed the Future: AgriLinks.
- ^ "Esoko website".
- Zeng, Yiwu; Jia, Fu; Wan, Li; Guo, Hongdong (24 July 2017). "E-commerce in agri-food sector: a systematic literature review". International Food and Agribusiness Management Review. 20 (4): 439–460. doi:10.22434/IFAMR2016.0156. ISSN 1559-2448.
- Hobbs et al. 2011. "International e-commerce: a solution to penetrating niche markets for food?" Estey Centre for Law and Economics in International Trade.
- Brugger 2011. "Mobile applications in agriculture." Syngenta Foundation.
- ^ Jouanjean, Marie-Agnes (15 February 2019). "Digital Opportunities for Trade in the Agriculture and Food Sectors". OECD Food, Agriculture, and Fisheries Papers, No. 122. OECD Food, Agriculture and Fisheries Papers. doi:10.1787/91c40e07-en.
- Lonie (2010). "Innovations in Rural and Agricultural Finance: M-PESA: Finding New Ways to Serve the Unbanked in Kenya". IFPRI: 2020 Vision for Food, Agriculture and the Environment.
- Hakobyan, Artavazd; Buyvolova, Anna; Meng, Yuan Ting; Nielson, David J. (1 January 2018). "Unleashing the Power of Digital on Farms in Russia - and Seeking Opportunities for Small Farms". World Bank. pp. 1–50.
- ^ Tarazi, Michael; Grossman, Jeremiah (1 June 2014). "Serving smallholder farmers: recent developments in digital finance". World Bank. pp. 1–16.
- Christine Martin; Nandini Harihareswara; Elizabeth Diebold; Harsha Kodali; Carrie Averch (2016). "Guide to the Use of Digital Financial Services in Agriculture" (PDF). USAID. Archived from the original (PDF) on 2018-09-06. Retrieved 2019-07-26.
- IFAD (2016). "Lessons learned: Digital financial services for smallholder households". International Fund for Agricultural Development. Archived from the original on January 20, 2022.
- Marulanda and the Bankable Frontier Associates (2015). "Colombia's Coffee Growers' Smart ID card: Successfully Reaching Rural Communities with Digital Payments" (PDF). Better Than Cash Alliance.
- Sitko, Nicholas J.; Bwalya, Richard; Kamwanga, Jolly; Wamulume, Mukata (2012). "Assessing the Feasibility of Implementing the Farmer Input Support Programme (FISP) Through an Electronic Voucher System in Zambia". Food Security Collaborative Policy Briefs 123210, Michigan State University, Department of Agricultural, Food, and Resource Economics.
- "AFCW3 Economic Update, Spring 2019: Digitizing Agriculture - Evidence from E-Voucher Programs in Mali, Chad, Niger, and Guinea". World Bank. 2019. doi:10.1596/31576. S2CID 242015695.
- Kärner, Ene (21 September 2017). "The Future of Agriculture is Digital: Showcasting e-Estonia". Frontiers in Veterinary Science. 4: 151. doi:10.3389/fvets.2017.00151. ISSN 2297-1769. PMC 5613108. PMID 28983486.
- Cook and McKay. "Top 10 Things to Know About M-Shwari." Consultative Group to Assist the Poor - Blog. 2 April 2015.
- "Winning in African agriculture". McKinsey. Retrieved 26 July 2019.
- "FarmDrive". farmdrive.co.ke. Retrieved 26 July 2019.
- Sylvester, Gerard (2018). "E-agriculture in action: drones for agriculture" (PDF). FAO and ITU.
- World Bank (27 June 2017). ICT in Agriculture (Updated Edition): Connecting Smallholders to Knowledge, Networks, and Institutions. The World Bank. doi:10.1596/978-1-4648-1002-2. hdl:10986/27526. ISBN 978-1-4648-1002-2.
- Poublanc, Christophe (26 October 2018). "Let's Get Digital: Un-Blocking Finance for Farmers in Senegal". USAID Feed the Future: Agrilinks Blog.
- Mitchell, Tara (2014). "Is Knowledge Power? Competition and Information in Agricultural Markets". The Institute for International Integration Studies Discussion Paper Series.
- Nakasone, Eduardo, ed. (2013). The Role of Price Information in Agricultural Markets: Experimental Evidence from Rural Peru. IFPRI.
- Thomas, Susan. "LOOP Mobile App Makes Farm to Market Linkages Easy". Digital Green. Retrieved 26 July 2019.
- ^ Schimmelpfennig (2016). "Farm Profits and Adoption of Precision Agriculture" (PDF). USDA Economic Research Service. Report no. 217.
- "Swarm robotics and the future of farming | AHDB". ahdb.org.uk. Archived from the original on 2021-09-28. Retrieved 2019-10-01.
- Acemoglu, D (1998). "Why Do New Technologies Complement Skills? Directed Technical Change and Wage Inequality". The Quarterly Journal of Economics. 113 (4): 1055–1089. doi:10.1162/003355398555838. hdl:1721.1/64397.
- Goldin and Katz (2008). The Race Between Education and Technology. Cambridge, MA: Belknap Press.
- Cole and Fernando (2012). "Mobile'izing Agricultural Advice: Technology Adoption, Diffusion and Sustainability". Harvard Business School Finance Unit. Research Paper No. 13-047.
- Demirguc-Kunt, Asli; Klapper, Leora; Singer, Dorothe; Ansar, Saniya; Hess, Jake (19 April 2018). The Global Findex Database 2017: Measuring Financial Inclusion and the Fintech Revolution. The World Bank. doi:10.1596/978-1-4648-1259-0. hdl:10986/29510. ISBN 978-1-4648-1259-0.
- Roscoe, Alexa; Hoffmann, Nathalie Ilona (1 October 2016). "Investing in women along agribusiness value chains". World Bank. pp. 1–65.
- Sandro Mendonça; Nuno Crespo; Nadia Simoes (2015). "Inequality in the Network Society: An Integrated Approach to ICT Access, Basic Skills, and Complex Capabilities". Telecommunications Policy. 39 (3–4): 192–207. doi:10.1016/j.telpol.2014.12.010.
- Quintini, Glenda; Nedelkoska, Ljubica (8 March 2018). "Automation, skills use and training". OECD Directorate for Employment, Labour, and Social Affairs - Employment, Labour, and Social Affairs Committee. OECD Social, Employment and Migration Working Papers. doi:10.1787/2e2f4eea-en.
- McKinsey & Company (2017). "Jobs lost, jobs gained: workforce transitions in a time of automation". McKinsey Global Institute.
- Maru, Berne, De Beer, Ballantyne, Pesce, Kalyesubula, Fourie, Addison, Collett, and Chaves 2018. "Digital and Data-Driven Agriculture: Harnessing the Power of Data for Smallholders." Global Forum on Agricultural Research and Innovation (GFAR); Global Open Data for Agriculture and Nutrition (GODAN); Technical Centre for Agricultural and Rural Cooperation (CTA).https://cgspace.cgiar.org/bitstream/handle/10568/92477/GFAR-GODAN-CTA-white-paper-final.pdf?sequence=3&isAllowed=y .
- Bongiovanni, R.; Lowenberg-Deboer, J. (1 August 2004). "Precision Agriculture and Sustainability". Precision Agriculture. 5 (4): 359–387. Bibcode:2004PrAgr...5..359B. doi:10.1023/B:PRAG.0000040806.39604.aa. ISSN 1573-1618. S2CID 13349724.
- ^ Eory, Vera; Barnes, Andrew; Gómez-Barbero, Manuel; Soto, Iria; Wal, Tamme Van der; Vangeyte, Jurgen; Fountas, Spyros; Beck, Bert; Balafoutis, Athanasios (2017). "Precision Agriculture Technologies Positively Contributing to GHG Emissions Mitigation, Farm Productivity and Economics". Sustainability. 9 (8): 1339. doi:10.3390/su9081339.
- European Parliament (2014). "Precision Agriculture: An Opportunity for EU Farmers - Potential Support with the CAP 2014-2020" (PDF). EU Parliament Directorate-General for Internal Policies, Policy Dept. B, Structural and Cohesion Policies: Agriculture and Rural Development.
- J.K. Berry; J.A. Detgado; R. Khosla; F.J. Pierce (2003). "Precision conservation for environmental sustainability". Journal of Soil and Water Conservation. 58 (6): 332–339.
- Katalin, Takács-György; Rahoveanu, Turek; Magdalena, Maria; István, Takács (1 January 2014). "Sustainable New Agricultural Technology – Economic Aspects of Precision Crop Protection". Procedia Economics and Finance. 1st International Conference 'Economic Scientific Research - Theoretical, Empirical and Practical Approaches', ESPERA 2013. 8: 729–736. doi:10.1016/S2212-5671(14)00151-8. ISSN 2212-5671.
- Gonzalez-Dugo, V.; Zarco-Tejada, P.; Nicolás, E.; Nortes, P. A.; Alarcón, J. J.; Intrigliolo, D. S.; Fereres, E. (1 December 2013). "Using high resolution UAV thermal imagery to assess the variability in the water status of five fruit tree species within a commercial orchard". Precision Agriculture. 14 (6): 660–678. Bibcode:2013PrAgr..14..660G. doi:10.1007/s11119-013-9322-9. ISSN 1573-1618. S2CID 14068322.
- V. Gonzalez-Dugo; P. Zarco-Tejada; E. Nicolás; P. A. Nortes; J. J. Alarcón; D. S. Intrigliolo; E. Fereres (2012). "Environmental and economic evaluation of N fertilizer rates in a maize crop in Italy: A spatial and temporal analysis using crop models". Biosystems Engineering. 113 (2): 103–111. Bibcode:2013PrAgr..14..660G. doi:10.1007/s11119-013-9322-9. S2CID 14068322.
- ^ Schieffer, J.; Dillon, C. (1 February 2015). "The economic and environmental impacts of precision agriculture and interactions with agro-environmental policy". Precision Agriculture. 16 (1): 46–61. Bibcode:2015PrAgr..16...46S. doi:10.1007/s11119-014-9382-5. ISSN 1573-1618. S2CID 9071060.
- "The role of digital in improving traceability and certification in the agricultural last mile". GSMA mAgri: Mobile for Development. 26 November 2018. Retrieved 26 July 2019.
- World Economic Forum and McKinsey & Company (2019). "Innovation with a Purpose: Improving Traceability in Food Value Chains through Technology Innovation" (PDF). World Economic Forum: System Initiative on Shaping the Future of Food.
- Friedlander, Blaine (10 May 2019). "Future cartons will track milk from farm to fridge". Cornell University. Retrieved 26 July 2019.
- Kent, Lampietti and Hasiner (2019). "Dead Branding Society: Is blockchain the death of food branding as we know it?". World Bank. Retrieved 26 July 2019.
- James Manyika; Sree Ramaswamy; Somesh Khanna; Hugo Sarrazin; Gary Pinkus; Guru Sethupathy; Andrew Yaffe (December 2015). "Digital America: A Tale of Haves and Have-Mores (Executive Summary)". McKinsey Global Institute. Archived from the original on 2019-05-05. Retrieved 2019-07-26.
- Shepherd, Mark; Turner, James A.; Small, Bruce; Wheeler, David (2018). "Priorities for science to overcome hurdles thwarting the full promise of the 'digital agriculture' revolution". Journal of the Science of Food and Agriculture. 100 (14): 5083–5092. doi:10.1002/jsfa.9346. ISSN 1097-0010. PMC 7586842. PMID 30191570.
- Lowenberg-DeBoer, James; Erickson, Bruce (2019). "Setting the Record Straight on Precision Agriculture Adoption". Agronomy Journal. 111 (4): 1552. Bibcode:2019AgrJ..111.1552L. doi:10.2134/agronj2018.12.0779. ISSN 0002-1962.
- Shepherd, Mark; Turner, James A.; Small, Bruce; Wheeler, David (2013). "Priorities for science to overcome hurdles thwarting the full promise of the 'digital agriculture' revolution". Journal of the Science of Food and Agriculture. 100 (14): 5083–5092. doi:10.1002/jsfa.9346. ISSN 1097-0010. PMC 7586842. PMID 30191570.
- "GitHub - InformationUpdates/SMARTFARM: Irrigation calculations for cultivating vegetables and fruits". GitHub. 14 May 2020.
- ^ Asian Development Bank (2018). "Internet plus agriculture: a new engine for rural economic growth in the People's Republic of China". Asian Development Bank. doi:10.22617/TCS189559-2. ISBN 978-92-9261-323-5.
- Arese Lucini; Okeleke; Tricarico (2016). "Analysis: Market size and opportunity in digitizing payments in agricultural value chains". GSMA Intelligence.
- ^ International Telecommunication Union as cited in Protopop and Shanoyan 2016. "Big Data and Smallholder Farmers: Big Data Applications in the Agri-Food Supply Chain in Developing Countries." International Food and Agribusiness Management Review Special Issue - Volume 19 Issue A, 2016.
- Xianqing Ji; Scott Rozelle; Jikun Huang; Linxiu Zhang; Tonglong Zhang (2016). "Are China's Farms Growing?" (PDF). China & World Economy. 24 (1): 41–62. doi:10.1111/cwe.12143. S2CID 35175511.
- Bukht and Heeks (2018). "Development Implications of Digital Economies: Digital Economy Policy in Developing Countries". Centre for Development Informatics Global Development Institute, SEED - Economic and Social Research Council. Paper no. 6.
- ^ Van Es and Woodard 2017. "Chapter 4: Innovation in Agriculture and Food Systems in the Digital Age." The Global Innovation Index 2017.
- Finistere Ventures, LLC (2018). "2018 Agtech Investment Review" (PDF).
- Acheampong (2019). "The Nature of Corporate Digital Agricultural Entrepreneurship in Ghana". Digital Entrepreneurship in Sub-Saharan Africa. Palgrave Studies of Entrepreneurship in Africa. pp. 175–198. doi:10.1007/978-3-030-04924-9_8. ISBN 978-3-030-04923-2. S2CID 169258652.
- Disrupt Africa (2018). "Agrinnovating for Africa: Exploring the African Agri-Tech Startup Ecosystem Report 2018".
- "Angola's go-to app for delivering live goats to your door". The Economist. 6 December 2018. ISSN 0013-0613. Retrieved 26 July 2019.
- ICT Update. No. 83. October 2016 https://cgspace.cgiar.org/bitstream/handle/10568/89782/ICT083E_PDF.pdf?sequence=2&isAllowed=y.
{{cite magazine}}
: Missing or empty|title=
(help) - Sherafat and Lehr (2017). "ICT-centric economic growth, innovation and job creation 2017" (PDF). International Telecommunication Union.
- Pollock, R. and Lämmerhirt, D. 2019. "Open data around the world: European Union." In T. Davies, S. Walker, M. Rubinstein, and F. Perini (Eds.), The state of open data: Histories and horizons(pp. 465-484). Cape Town and Ottawa: African Minds and International Development Research Centre.
- Aysha Fleming; Emma Jakku; Lilly Lim-Camacho; Bruce Taylor; Peter Thorburn (2018). "Is big data for big farming or for everyone? Perceptions in the Australian grains industry". Agronomy for Sustainable Development. 38 (24). doi:10.1007/s13593-018-0501-y.
- ^ Wiseman, Leanne; Sanderson, Jay; Zhang, Airong; Jakku, Emma (2019). "Farmers and their data: An examination of farmers' reluctance to share their data through the lens of the laws impacting smart farming". NJAS - Wageningen Journal of Life Sciences. 90–91: 100301. doi:10.1016/j.njas.2019.04.007. hdl:10072/385974.
- ^ "DLG e.V. - Digital Agriculture - Opportunities. Risks. Acceptance". www.dlg.org. Retrieved 26 July 2019.
- Lesser 2014; Orts and Spigonardo 2014; Sonka 2014; Van't Spijker 2014 — all as cited in Wolfert, Ge, Verdouw, and Bogaardt. 2017. "Big data in smart farming – A review." Agricultural Systems, Volume 153, pp. 69-80.
- European Parliamentary Research Service 2017. "Precision agriculture in Europe: Legal, social and ethical considerations." European Parliament Think Tank.13 November 2017.
- GODAN as cited in Carolan, Michael (2017). "Publicising Food: Big Data, Precision Agriculture, and Co-Experimental Techniques of Addition". Sociologia Ruralis. 57 (2): 135–154. doi:10.1111/soru.12120..
- "Business Secretary calls for new tech revolution in agriculture". GOV.UK. Retrieved 26 July 2019.
- Michalopoulos, Sarantis (30 October 2018). "Greek plan to digitise agriculture wins EU approval". euractiv.com. Retrieved 26 July 2019.
- "National Institute of Food and Agriculture". nifa.usda.gov. Retrieved 11 August 2021.
- "NSF - National Science Foundation". nsf.gov. Retrieved 11 August 2021.
- "Home". Internet of Things for Precision Agriculture. Retrieved 11 August 2021.
- "COALESCE". Iowa State University. Retrieved 11 August 2021.
- "Digital Agriculture: feeding the future". Project Breakthrough. Retrieved 10 December 2018.
- Blahe, Wahyu (10 November 2019). "Digital Farmers". petanidigital.id. Retrieved 12 December 2020.